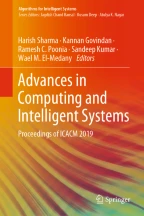
Deep learning is extremely popular among data scientists and analysts due to its proven accuracy in recognizing speech, image processing, and Big Data analytics. Data is growing at exponential rate due to sensor networks and communications. It is analyzed that 90% data was generated during last 2 years and data will be increased at this pace in forthcoming years also. Deep learning has become more significant as it can be used for large scale of unlabeled data. The increase in number of hidden nodes with the sheer size of data resists learning. Deep learning with new algorithmic approaches is able to learn large-scale unlabeled data with less feature engineering. Big Data can be better analyzed by deep learning. In this paper, the focus is on new algorithms and techniques of deep learning which can provide better accuracy for large-scale complex and unstructured data.
This is a preview of subscription content, log in via an institution to check access.
Access this chapter
Subscribe and save
Springer+ Basic
€32.70 /Month
- Get 10 units per month
- Download Article/Chapter or eBook
- 1 Unit = 1 Article or 1 Chapter
- Cancel anytime
Buy Now
Price includes VAT (France)
eBook EUR 117.69 Price includes VAT (France)
Softcover Book EUR 158.24 Price includes VAT (France)
Hardcover Book EUR 158.24 Price includes VAT (France)
Tax calculation will be finalised at checkout
Purchases are for personal use only
Similar content being viewed by others
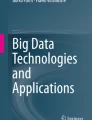
Chapter © 2016
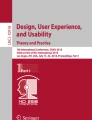
Deep Learning Model and Its Application in Big Data
Chapter © 2018
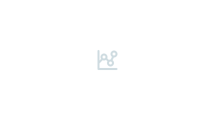
Deep learning applications and challenges in big data analytics
Article Open access 24 February 2015
References
- LeCun, Y., Bengio, Y., & Hinton G. (2015). Deep learning. Nature, 521(7553), 436–444. Google Scholar
- Raina, R., Madhavan, A., & Ng, A. Y. (2009). Large-scale deep unsupervised learning using graphics processors. In Proceedings of the 26th Annual International Conference on Machine Learning (pp. 873–880). Google Scholar
- Cireşan, D. C., Meier, U., Gambardella, L. M., & Schmidhuber, J. (2010). Deep, big, simple neural nets for handwritten digit recognition. Neural Computation, 22(12), 3207–3220. Google Scholar
- Zhang, Q., Yang, L. T., Chen, Z., & Li, P. (2018). A survey on deep learning for big data. Information Fusion, 42, 146–157. Google Scholar
- Hinton, G. E., & Salakhutdinov, R. R. (2006). Reducing the dimensionality of data with neural networks. Science, 313(5786), 504–507. Google Scholar
- Hinton, G. E. (2012). A practical guide to training restricted Boltzmann machines. In Neural networks: Tricks of the trade (pp. 599–619). Springer, Berlin, Heidelberg. Google Scholar
- Chen, M., Mao, S., & Liu, Y. (2014). Big data: A survey. Mobile Networks and Applications, 19(2), 171–209. Google Scholar
- Fahad, A., Alshatri, N., Tari, Z., Alamri, A., Khalil, I., Zomaya, A. Y., et al. (2014). A survey of clustering algorithms for big data: Taxonomy and empirical analysis. IEEE Transactions on Emerging Topics in Computing, 2(3), 267–279. Google Scholar
- Cui, X., Zhu, P., Yang, X., Li, K., & Ji, C. (2014). Optimized big data K-means clustering using MapReduce. The Journal of Supercomputing,70(3), 1249–1259. Google Scholar
- Sharma, K. P., Poonia, R. C., & Sunda, S. (2018). Real time location tracking map matching approaches for road navigation applications. Data Intensive Computing Applications for Big Data, 29, 414. Google Scholar
- Gandomi, A., & Haider, M. (2015). Beyond the hype: Big data concepts, methods, and analytics. International Journal of Information Management,35(2), 137–144. Google Scholar
- Munjal, P., Kumar, S., Kumar, L., & Banati, A. (2017). Opinion dynamics through natural phenomenon of grain growth and population migration. In Hybrid intelligence for social networks (pp. 161–175). Springer, Cham. Google Scholar
- Munjal, P., Kumar, L., Kumar, S., & Banati, H. (2019). Evidence of Ostwald Ripening in opinion driven dynamics of mutually competitive social networks. Physica A: Statistical Mechanics and Its Applications. Google Scholar
- Cambria, E., Rajagopal, D., Olsher, D., & Das D. (2013). Big social data analysis. Big Data Computing, 13, 401–414. Google Scholar
- Guellil, I., & Boukhalfa, K. (2015). Social big data mining: A survey focused on opinion mining and sentiments analysis. In International Symposium on Programming and Systems (ISPS) (pp. 1–10). IEEE. Google Scholar
- Bello-Orgaz, G., Jung, J. J., & Camacho, D. (2016). Social big data: Recent achievements and new challenges. Information Fusion, 28, 45–59. Google Scholar
- Chen, X. W., & Lin, X. (2014). Big data deep learning: Challenges and perspectives. IEEE Access,2, 514–525. Google Scholar
- Al-Jarrah, O. Y., Yoo, P. D., Muhaidat, S., Karagiannidis, G. K., & Taha, K. (2015). Efficient machine learning for big data: A review. Big Data Research, 2(3), 87–93. Google Scholar
- Deng, L., Yu, D., & Platt, J. (2012). Scalable stacking and learning for building deep architectures. In Proceedings of IEEE ICASSP (pp. 2133–2136). Google Scholar
- Dean, J., Corrado, G., Monga, R., Chen, K., Devin, M., Mao, M., et al. (2012). Large scale distributed deep networks. In Proceedings of Advances in Neural Information Processing Systems (pp. 1223–1231). MIT. Google Scholar
- Zhang, K., & Chen, X. W. (2014). Large-scale deep belief nets with mapreduce. IEEE Access, 2, 395–403. Google Scholar
- Sohangir, S., Wang, D., Pomeranets, A., & Khoshgoftaar, T. M. (2018). Big data: Deep learning for financial sentiment analysis. Journal of Big Data,5(1), 3. Google Scholar
- Abadi, M., Barham, P., Chen, J., Chen, Z., Davis, A., Dean, J., et al. (2016). Tensorflow: A system for large-scale machine learning. In 12th Symposium on Operating Systems Design and Implementation (pp. 265–283). Google Scholar
Author information
Authors and Affiliations
- Punjabi University, Patiala, India Gourav Bathla & Himanshu Aggarwal
- Thapar University, Patiala, India Rinkle Rani
- Gourav Bathla